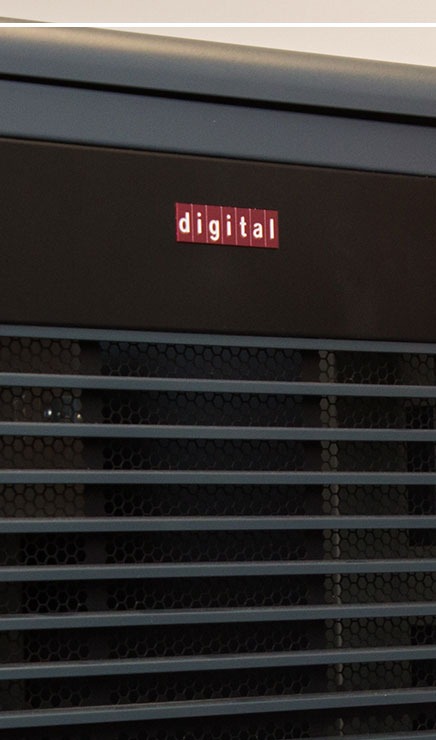
Artificial Intelligence Laboratory
Visionary information systems research to address pressing societal challenges.
Founded and led by UA Regents’ Professor Hsinchun Chen, the Eller Artificial Intelligence Laboratory is the world’s only AI lab or center within a business school.
In this unique setting, and under visionary stewardship, the lab has become a distinguished academic force making significant contributions to our understanding of health informatics, security informatics, business intelligence and knowledge management.
Featured Research: Cybersecurity
As a global society, we increasingly rely on a complex, fragile and vulnerable cyberspace. Through cybersecurity related projects, research and education, the AI Lab aims to address these societal challenges, employing cutting-edge scientific and engineering methods. Through projects like the AZSecure Cybersecurity Program—which produces well-trained, expert information security professionals—and the Hacker Assets Portal, the AI Lab is building invaluable understanding and developing students into major assets in the cybersecurity workforce.
Learn More About the AI Lab's Cybersecurity Research and Education
A Broad Reach
Research findings from the Artificial Intelligence Lab have been featured in Science, The New York Times, Los Angeles Times, Business 2.0, The Police Chief, The Washington Post, Time Magazine Global Business Supplement, Newsweek, ABC News and The Boston Globe, among others.
Our Research
Research Projects
Through sophisticated information systems research, the AI Lab has made significant contributions in machine learning, visualization, knowledge management and other critical areas. Explore our groundbreaking current research projects—and past projects that shaped our understanding of the world.
Resources
Browse resources like the H Index for Management Information Systems, articles and other publications stemming from AI Lab research, the AZSecure Cybersecurity Fellowship Program and even helpful templates for producing research papers and publications.
Publications of the AI Lab
The Eller AI Lab has been the birthplace of numerous papers in highly respected professional and scientific publications. Browse our faculty and student publications in journals like MIS Quarterly, Journal of Management Information Systems, Journal of Biomedical Informatics and countless others.
Photo Galleries
Click an image to view in larger format:
News
Events
No upcoming events are available at this time.
Contact Us
By Phone
520-621-6219 office
By Email
General Inquiries
brandig@email.arizona.edu
By Mail
McClelland Hall 430
The University of Arizona
P.O. Box 210108
Tucson, AZ 85721-0108